Artificial Intelligence (AI) is a broad field aiming to create machines that can mimic human intelligence. Machine Learning (ML) is a subset of AI focused on enabling machines to learn from data.
Artificial Intelligence encompasses a wide range of technologies designed to perform tasks that typically require human intelligence, such as reasoning, problem-solving, and understanding natural language. Machine Learning, on the other hand, specifically refers to algorithms and statistical models that allow computers to learn and make decisions based on data.
While AI aims to create intelligent systems that can perform a variety of tasks, ML focuses on developing techniques that enable machines to learn and improve from experience. This distinction makes AI a broader concept, with ML being one of its key components.
Ai And Machine Learning: Clearing The Confusion
Artificial Intelligence (AI) is a broad field. It involves creating machines that can mimic human intelligence. AI includes various technologies like expert systems, natural language processing, and robotics.
Machine Learning (ML) is a subset of AI. It focuses on enabling machines to learn from data. ML uses algorithms to identify patterns and make decisions. AI is the big picture, while ML is one part of that picture.
AI research began in the 1950s. Early AI aimed to create machines that could think like humans. These early systems used rules and logic to make decisions.
Machine Learning became popular in the 1980s. Researchers developed algorithms that could learn from data. This approach was different from early AI, which relied on hard-coded rules.
Defining The Territory: Ai In A Nutshell
Artificial Intelligence (AI) is a broad field of computer science. It aims to create machines that can mimic human intelligence. AI encompasses various technologies and techniques. It includes machine learning (ML), but also other areas like natural language processing and robotics.
AI aims to build systems that can perform tasks which typically require human intelligence. These tasks can range from simple problem-solving to complex decision-making. AI systems can learn, adapt, and improve over time. They analyze vast amounts of data to provide useful insights.
AI applications go beyond just machine learning. They can include robotics, where AI controls physical robots. AI is also used in natural language processing to understand human speech. Another example is computer vision, where AI helps in image recognition. Each of these applications demonstrates the broad scope of AI.
Zooming In On Machine Learning
Machine Learning (ML) uses data to build models that can make predictions. These models improve over time without explicit programming. Algorithms in ML learn patterns from data. Then, they use these patterns to make decisions or predictions. Data is the key component. Better data leads to better models.
Supervised learning and unsupervised learning are two main types of ML. Supervised learning uses labeled data. It predicts outcomes based on input-output pairs. Unsupervised learning finds hidden patterns in data without labels.
Predictive maintenance in factories uses ML to forecast equipment failures. This helps in reducing downtime and saving costs. Dynamic pricing in travel adjusts ticket prices based on demand. ML helps airlines and hotels optimize revenue.
Fraud detection in finance uses ML to spot unusual transactions. This protects customers from fraud. Retail demand forecasting helps stores manage inventory. ML predicts which products will sell and in what quantities.
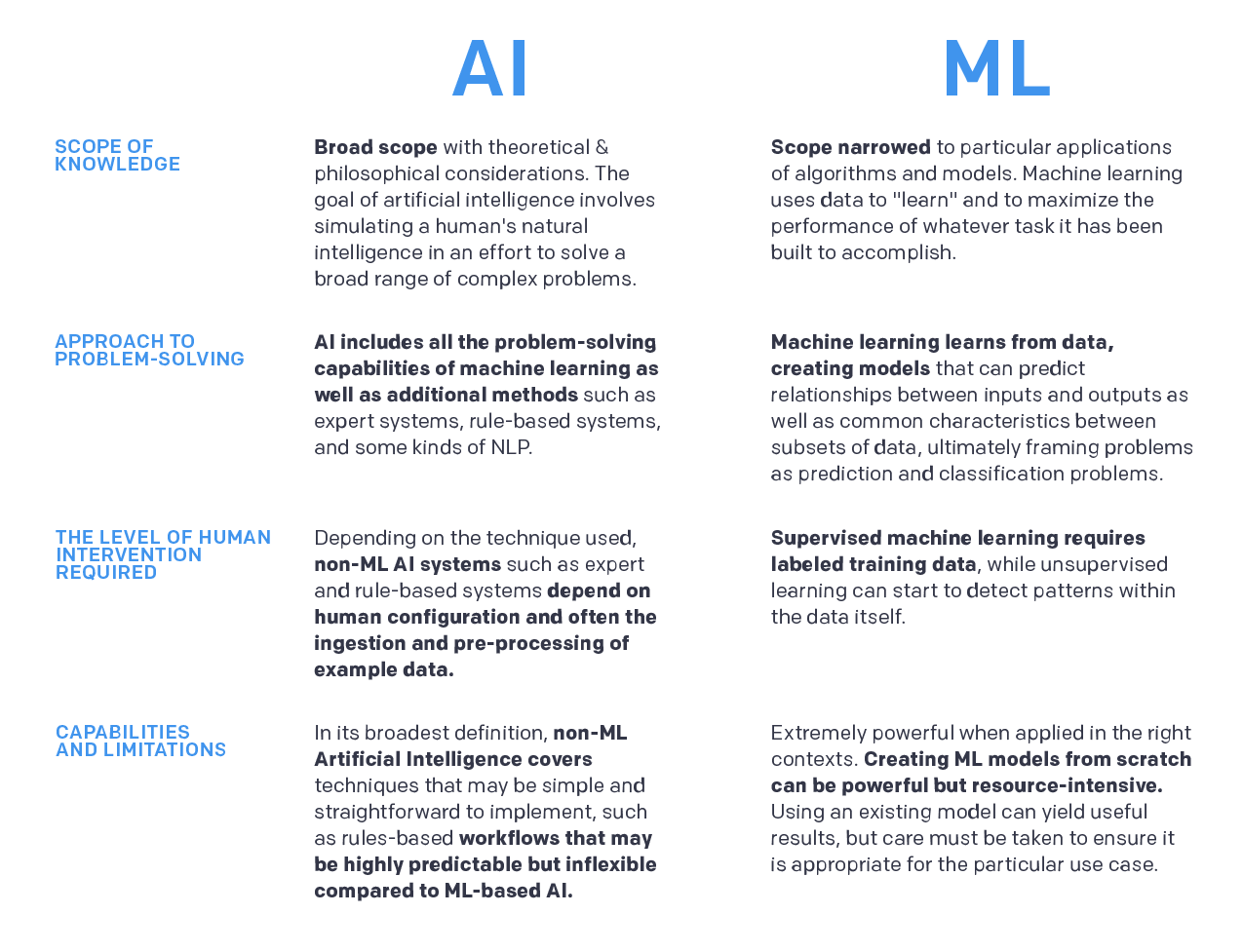
Credit: gigster.com
How Ai And Ml Intersect And Diverge
Machine Learning (ML) is a subset of Artificial Intelligence (AI). AI is a broad field aiming to create machines that can perform tasks requiring human intelligence. These tasks include reasoning, learning, and problem-solving. ML focuses on enabling machines to learn from data. This involves training algorithms to improve performance over time.
AI aims to create systems that can perform a wide range of tasks. These tasks often mimic human capabilities. Examples include speech recognition and language translation. Machine Learning, on the other hand, aims to optimize performance based on data. This involves techniques like supervised learning and unsupervised learning. The main goal is to improve accuracy and make predictions.
The Role Of Data: Ai Vs. Ml
Artificial Intelligence can interpret large sets of data to make decisions. AI systems analyze data to find patterns and insights. Complex algorithms help AI understand and predict outcomes. AI mimics human intelligence to solve problems. AI applications include language translation and image recognition.
Machine Learning excels at pattern recognition within data sets. ML algorithms learn from historical data to make predictions. Supervised learning uses labeled data for training. Unsupervised learning finds hidden patterns in data. Reinforcement learning improves decisions based on feedback.
Decision-making: Contrasting Ai And Ml
AI systems can make decisions based on data and predefined rules. These rules help AI to analyze situations and choose actions. AI can work autonomously without human intervention. It mimics human decision-making processes to solve complex problems.
Machine Learning (ML) focuses on learning from data. ML algorithms require training with large datasets. These algorithms improve over time through experience. Human experts set up and optimize these systems. ML adapts to new data without being explicitly programmed.
Impact On Careers: Ai And Ml Specializations
AI offers many exciting job roles. AI researchers work on new algorithms and theories. AI engineers build and deploy AI models. Data scientists analyze data to find patterns. Machine learning engineers focus on creating systems that learn from data. AI product managers oversee AI projects from start to finish. AI consultants help businesses integrate AI solutions. AI ethicists ensure AI technologies are used responsibly.
ML specialists have diverse career paths. ML engineers develop algorithms that improve over time. Data analysts interpret complex data and provide insights. Research scientists explore new methods in machine learning. Business intelligence developers create tools for data-driven decisions. Big data engineers handle large datasets and optimize ML models. Deep learning engineers specialize in neural networks and complex models. ML operations (MLOps) engineers streamline the deployment of ML systems.
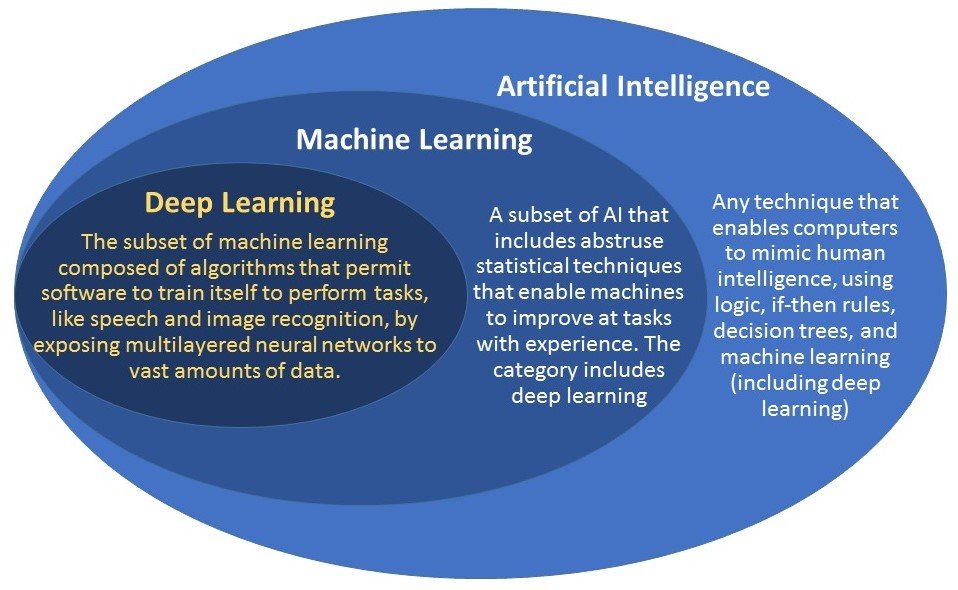
Credit: www.pistoiaalliance.org
Future Trajectories: Ai And Ml Evolutions
AI has seen rapid growth in recent years. Natural language processing is now more advanced. AI systems can understand and generate human language. Computer vision has also improved. AI can now recognize images with high accuracy. Autonomous vehicles are another area of progress. Cars can drive themselves with minimal human input. These advancements make AI more versatile.
Machine Learning has made significant strides too. Supervised learning helps in making predictions based on data. Unsupervised learning finds patterns without labeled data. Reinforcement learning is crucial for robotics. Machines learn from trial and error to improve. Deep learning has revolutionized ML. It mimics the human brain to solve complex problems. These innovations make ML more powerful.

Credit: alrayyantv-wp2.alrayyantv-ase.p.azurewebsites.net
Frequently Asked Questions
Are Machine Learning And Ai The Same?
No, machine learning and AI are not the same. AI is the broader concept of machines simulating human intelligence. Machine learning is a subset of AI focused on systems learning from data.
What Is An Example Of Ai Vs Ml?
AI powers virtual assistants like Siri, while ML predicts product recommendations on e-commerce sites.
What Are Two Differences Each Between Artificial Intelligence And Machine Learning?
Artificial Intelligence (AI) aims to create systems that mimic human intelligence. Machine Learning (ML) focuses on learning from data. AI includes a broader range of technologies, while ML is a subset of AI. AI can function without ML, but ML requires AI principles.
Conclusion
Understanding the difference between AI and machine learning is crucial in the tech world. AI aims to create smart machines. Machine learning, a subset, focuses on data-driven learning. Both fields offer exciting possibilities and advancements. Stay curious and continue exploring this fascinating technology.
Embrace the future of intelligent systems and their potential to transform our lives.