Supervised machine learning uses labeled data to train models. Unsupervised machine learning finds patterns in data without labels.
Supervised machine learning involves using a labeled dataset to teach the model how to predict outcomes. It is often used in tasks like classification and regression, where the system learns from examples. Unsupervised machine learning, on the other hand, identifies hidden patterns in data without predefined labels.
This approach is useful in clustering, association, and dimensionality reduction. Both techniques are fundamental in data science, offering distinct ways to analyze and interpret complex datasets. Understanding the differences helps in choosing the right method for specific applications, ensuring accurate and efficient data analysis.
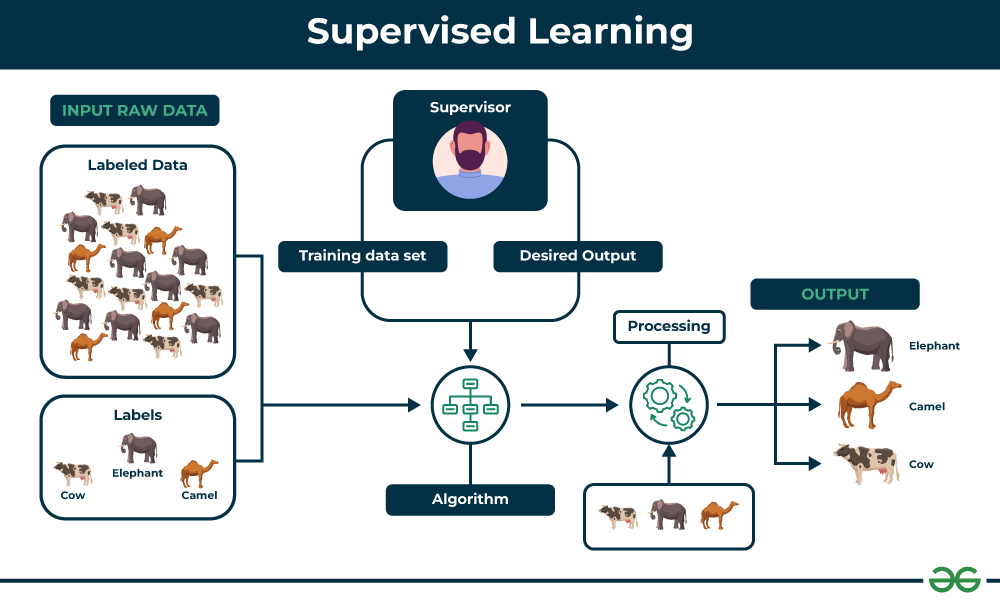
Credit: www.geeksforgeeks.org
Introduction To Machine Learning
Artificial Intelligence has grown fast. Machines can now think and learn. This progress amazes many people. Machine Learning is a part of AI. It helps machines learn from data. AI is now in many daily tools.
Machine Learning has important ideas. Supervised learning uses labeled data. This helps machines predict the future. Unsupervised learning works with unlabeled data. It finds hidden patterns. Both methods are powerful. Each has its own uses.

Credit: www.v7labs.com
Supervised Learning Explained
Supervised learning needs labeled data. Each data point has a label. The model learns by seeing the labels. This helps the model make accurate predictions. Labeled data acts as a guide for the model. It shows the model what to learn.
Common algorithms include Linear Regression and Decision Trees. Support Vector Machines are also popular. These algorithms solve many problems. They help in spam detection and image recognition. They are also used in medical diagnosis.
Unsupervised Learning Demystified
Unsupervised learning works without labels. It finds patterns in data. Computers group similar items together. This is done without any help. Data is not labeled or categorized.
Clustering is grouping similar data points. It helps in identifying natural clusters. Items in a cluster are alike. Association finds rules in data. It shows how items are related. Both are key techniques in unsupervised learning.
Comparing Supervised And Unsupervised Learning
Supervised learning needs labeled data. This means that each data point has a known outcome. Preparing data for supervised learning can be time-consuming. It requires human effort to label the data. This ensures accuracy in training the model. Unsupervised learning does not need labeled data. The algorithm finds patterns on its own. This makes it faster to prepare data. But, results may be less accurate.
Supervised learning is good for tasks like classification and regression. It can predict outcomes and categorize data. It works well for spam detection, image recognition, and sales forecasting. Unsupervised learning is useful for clustering and association. It helps in market segmentation, anomaly detection, and customer behavior analysis. Supervised learning is often easier for beginners. Unsupervised learning may require more advanced understanding.
Hybrid Approaches: Semi-supervised And Reinforcement Learning
Hybrid approaches mix supervised and unsupervised methods. These methods use labeled and unlabeled data. This combination provides more robust models. Semi-supervised learning uses a small amount of labeled data. The rest is unlabeled. This saves time and resources. Reinforcement learning is another hybrid approach. It learns from rewards and penalties. This method is useful in dynamic environments. Combining these techniques improves accuracy. It also enhances adaptability. Hybrid approaches can handle complex tasks better.
Hybrid learning is used in many fields. Self-driving cars are one example. These cars use supervised and reinforcement learning. They recognize objects and make decisions. Medical diagnosis is another area. Doctors use hybrid models to analyze patient data. This helps in accurate predictions. Fraud detection also benefits. Banks use these models to identify suspicious activities. Hybrid learning improves efficiency and accuracy. It also reduces human error.
Challenges And Considerations
Understanding the challenges and considerations in supervised vs unsupervised machine learning is crucial for effective model selection and implementation. Factors such as data labeling, algorithm complexity, and performance metrics play significant roles in determining the best approach.
Data Quality And Quantity
Data quality is very important in machine learning. Good data makes better models. Bad data can lead to poor results. Large amounts of data are often needed. This is especially true for supervised learning. Clean data is also very important. Noise and errors in data can confuse the model. Collecting good data can be hard and expensive. Not all data is useful. Some data may need to be removed.
The Ethical Implications Of Machine Learning
Machine learning can raise ethical issues. Bias in data can lead to unfair outcomes. Privacy is another concern. People’s data must be kept safe. Transparency is important. People should know how decisions are made. Accountability is also key. Someone must be responsible for the outcomes. Ethical guidelines help address these issues.
Future Trends In Machine Learning
New algorithms are making machine learning smarter. These algorithms learn faster and use less data. This means better results in less time. Deep learning is one such technique. It is being improved every day. Smaller models are now as powerful as bigger ones. This saves both time and money.
Unsupervised learning is getting more attention. It helps find patterns in data without labels. This means it can discover hidden insights. Businesses use it to understand customers better. It can also detect fraud in real-time. Unsupervised learning is crucial for big data. It makes sense of large amounts of information. This makes it a powerful tool for the future.
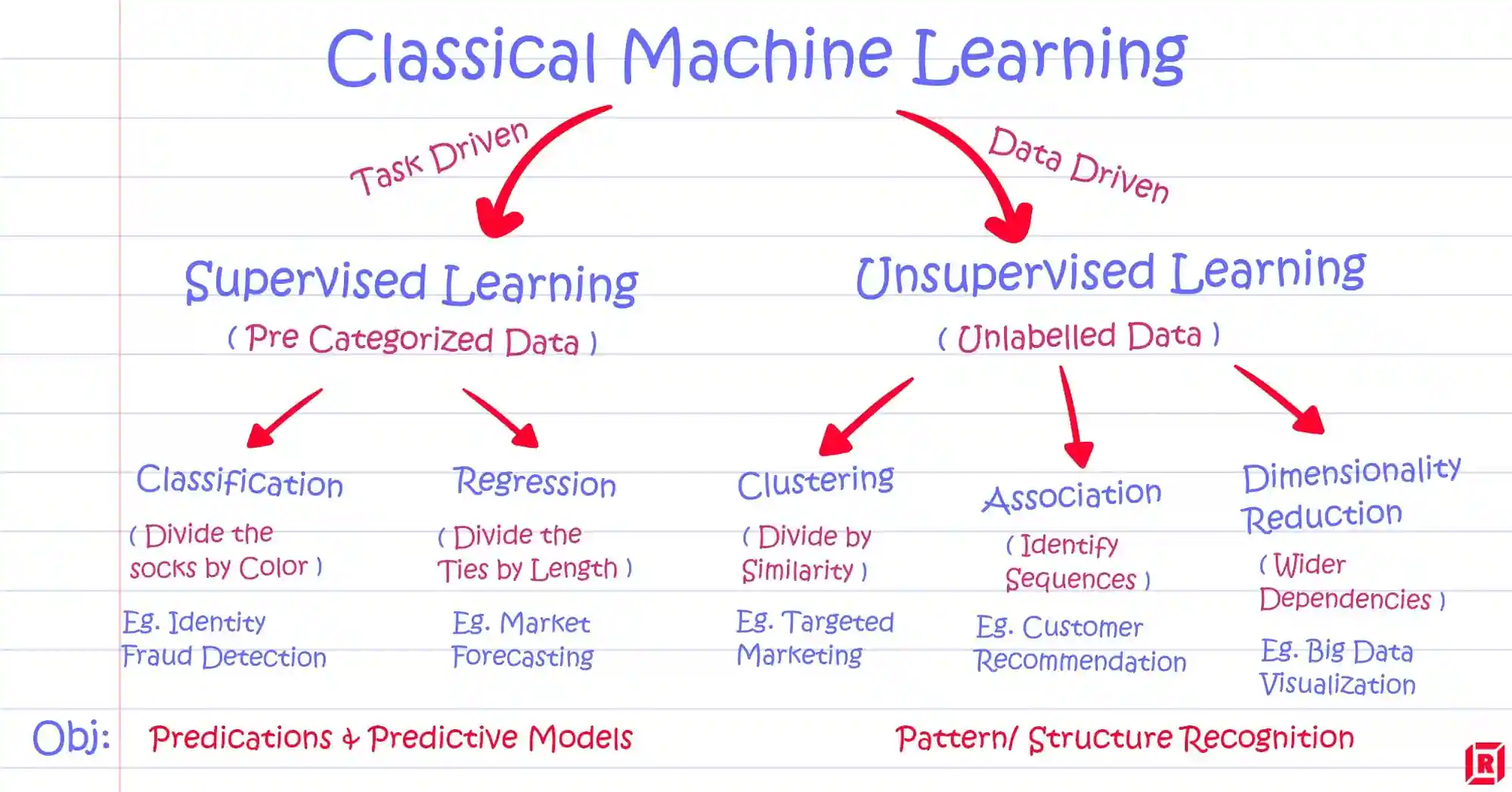
Credit: www.labellerr.com
Conclusion: The Continuous Evolution Of Machine Learning
Machine learning transforms industries like healthcare, finance, and retail. It helps in predicting trends, improving customer experiences, and automating tasks. Hospitals use it to diagnose diseases early. Banks detect fraud faster and more accurately. In retail, personalized shopping experiences increase sales. Machine learning also impacts society by enhancing public safety and transportation. Smart cities use data to manage resources efficiently. Self-driving cars reduce traffic accidents and emissions. The benefits are endless.
The future is bright for machine learning. New algorithms and better hardware make it more powerful. AI ethics and regulations will guide its use. Collaboration between humans and machines will become seamless. Education will adapt to teach these new skills. Businesses will need to stay updated. Research in this field will continue to grow. We are just at the beginning of this technological revolution.
Frequently Asked Questions
What Is The Difference Between Supervised And Unsupervised Learning?
Supervised learning uses labeled data for training, while unsupervised learning uses unlabeled data to find patterns. Supervised models predict outcomes, whereas unsupervised models identify structures.
What Is An Example Of Supervised Learning?
An example of supervised learning is email spam detection. The algorithm learns from labeled emails to classify new ones as spam or not.
Is Cnn Supervised Or Unsupervised?
CNN is a supervised learning algorithm. It uses labeled data to train and make predictions.
What Is An Example Of Supervised Unsupervised And Reinforcement Learning?
Supervised learning example: Email spam detection. Unsupervised learning example: Customer segmentation. Reinforcement learning example: Training a robot to walk.
Conclusion
Supervised and unsupervised machine learning each have unique strengths. Choosing the right approach depends on your data and goals. Supervised learning excels with labeled data, while unsupervised learning finds hidden patterns. Understanding both can significantly impact your machine learning projects.
Keep exploring to harness their full potential.